In this story, we explore Planisware's journey towards applying Artificial Intelligence (A.I.) to Project Portfolio Management.
What has changed today that makes Artificial Intelligence and Machine Learning viable techniques in the Project Portfolio world?
And what are the main innovative use cases where A.I. can be deployed today?
- From Bust to Boom: A.I. applied to PPM
Estimation and user enablement - why A.I. now makes sense in the PPM world - Offer your users a Smart Assistant
Empower your users and reduce data issues with chatbots - Interact with projects on the go
Advanced interaction and project updates from anywhere - Leverage project data with Machine Learning
Train an estimation model for data-driven forecasting
From Bust to Boom: Artificial Intelligence applied to Project Portfolio Management
Pierre smiles as he gazes out the conference room window, over the Paris skyline at the Eiffel Tower. “Most of Planisware’s founders, and myself, we started our career working on A.I. (Artificial Intelligence) projects in the 80s.” Now CEO of Planisware, his trim beard flecked with gray, Pierre Demonsant vividly remembers the first “winter of A.I.,” when many ambitious A.I. projects failed to deliver on their promises. “Those struggles are why I changed course to start working on Project Portfolio Management in the early 90s.”
“Three years ago, I again started exploring A.I. ,” explains Pierre. “Today, we can access very large data sets with more computational power -- which means we have a chance now to successfully address more use cases,” he explains. “But I have learned prudence, and prefer to only talk about projects that we have delivered.”
Planisware started its journey toward integrating AI into its main products by exploring whether machine learning could help project leaders better assess expected project durations and cost estimates early on.
Paul, a slim, blond figure in his thirties, is managing the Planisware Machine Learning effort in R&D. Paul recalls. “We started our work by asking our customers to share their database with us. The idea was to investigate what would be the most suitable model to apply to the data, and then train the model afterwards.” Planisware customers’ databases often have millions of objects - the strategy seemed sound.
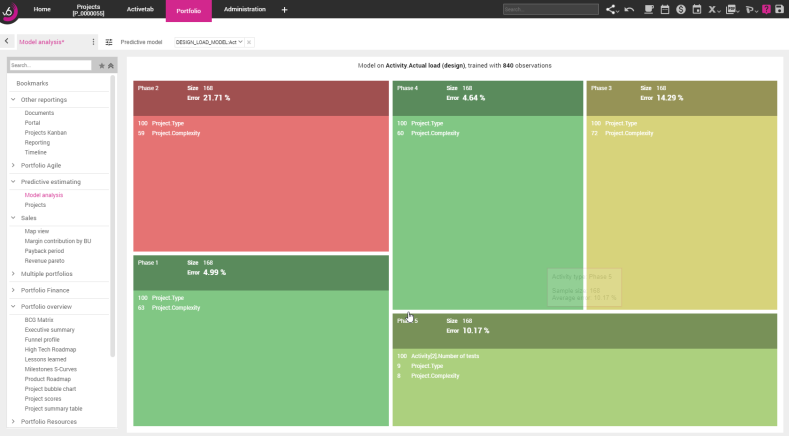
Above: Predictive Estimation model applied to the Company Portfolio.
“But reality kicked in,” continues Paul. “Data quality was uneven. It was difficult to have good results across the board. In some areas we could get some results, but in others it was very difficult to get a reliable forecast,” Paul quietly tells us. “It came to us as a bit of a surprise, but in the end, it turned out that it was because we wanted to use the data in a multifaceted way -- not how you typically use it, because people tend to focus on specific metrics.”
“Even when users control their projects and follow corporate standards, data quality can suffer very quickly” explains Franck Lafitte. Franck, Head of Planisware Consulting, is a tall man in his fifties, with an amused air about him. “Even with only 10 users, mistakes are made almost daily and can be difficult to spot. This issue gets compounded as the number of users gets higher.”
”There are so many mistakes that can be made daily,” continues Franck. “Like re-using templates that don’t fit a project. Or costs or labor reported against the wrong task. You might have good data when it is used for computing key performance indicators.”
Franck pauses a bit before cautioning, “However, implementation choices, like the level of granularity of cost management, could also limit how you can use that data for forecasting.”
Offer your Users a Smart Assistant
“To go any further, we had to find a way to help our customers with data quality,” says Paul. “So, what about using A.I. to help them? We started to think about an always-on A.I. presence next to the users as a great way to help ensure data quality.”
“That seemed worth trying,” notes Pierre. He explains that, while bots used to rely on a rigid set of rules and scripted answers, they are now able to interact in a more natural, less structured fashion. “Advances in Natural Language processing are the key,” he says. “Processing normal human speech or text, computers can now understand context and make useful connections. Conversational bots - or chatbots - have become more accepted to interact with a service.”
“Chatbots are mostly just used in e-commerce today,” Paul says. “However, they can have their logic repurposed to serve as an always-on assistant for things like user on-boarding, guided tours of screens, or tool tips in context, and thus be of help with data quality.”
Good data quality depends heavily on users understanding the meaning of key processes and the significance of the data they enter. A chatbot can be a valuable companion, reminding users how to deal with specific process and what kind of data is required.
Above. Planisware Chatbot: A brief demonstration. Request and update information directly through chat.
Interact with your Projects on the Go
Paul explains that, while working on the initial chatbot project to provide guidance to users, the product team soon had the idea of using the bot to update and engage with projects. “This scenario surfaced very quickly. However our language, when it intersects with the reality of projects, is very difficult for a machine to understand.
“It starts with project names, which are frequently common words or words that the bot might interpret as actionable. We had to develop a completely new approach to deal with truncated or misleading statements,” says Paul.
The goal was to make the bot engage in interactive conversation – to respond, not just by doing as told, but by interpreting and asking for clarification. To achieve this, the bot needed to fully understand natural phrasing, which can vary immensely among users, without relying on the use of specific keywords.
“We really had to keep the interaction fluid,” Paul adds. “Above all we had to adapt as closely as we could to the language of our users. We learned very early on that we had to preserve flexibility to let the bot adapt to local use of words.”
Leverage Project Data with Machine Learning
“I was an early customer who Planisware asked about using data for machine learning. That was two years ago,” Jeff says. ”And after a few months, I went back to Planisware to try to understand what they got out of my input . . .” Jeff tells his story to an eager audience in a packed room, at the Planisware User Summit 2019.
Jean-Philippe Castells - or just Jeff to friends - is an IT Director with UCB in Belgium, and his enthusiasm for A.I. initiatives is infectious. The audience listens intently as Jeff tells how UCB, a major pharmaceutical company, has always understood that data science is the future. This was the year that Planisware A.I. leveraging financial data pre-populated the budget. “A.I. is now mature enough to move forward, and we will have more and more data coming out of AI and machine learning”.
Paul, joining Jeff onstage, explains how Planisware machine learning tools provide a simple mechanism to train a model with data. Once trained, the model can generate data-driven estimates for various aspects of project activities: durations, resource consumption, costs, and more. Paul stresses that although the system employs advanced mathematics and learning techniques, the machine-learning feature itself remains very easy to use. “That’s something that took us some time to figure out how to do,” explains Paul.
An early result was that the Planisware Predictive Analysis model could quickly spot outliers and data quality issues in the database. “It’s like the defects are jumping at your face,” jokes Jeff in front of the audience. Users can then quickly address those issues and start educating the user base with specific, targeted actions.
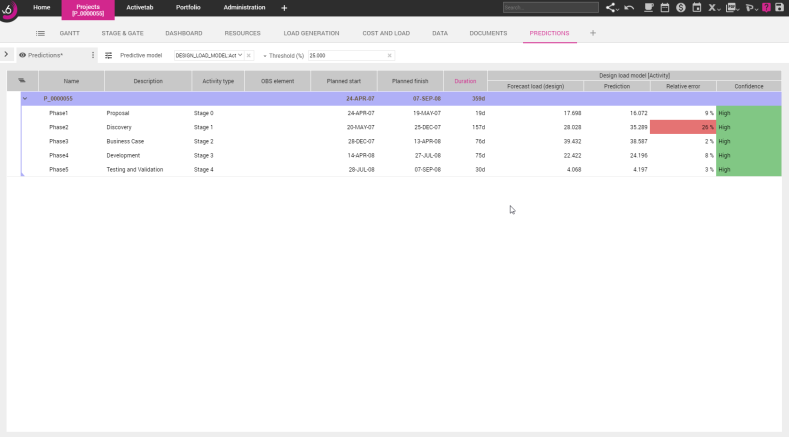
Above: Spotting outliers: How reliable is the predictive model?
Another application of A.I. in Planisware is Predictive Estimation, which can identify the drivers behind estimating trends and data points, in order to confirm and to gain fresh insight into what is really driving project activities. Understanding of the drivers enables accurate forecasting of project cost, duration, and resource levels, either immediately upon project creation to help project leaders build business cases or to provide up-to-date estimates at completion once the project is underway.
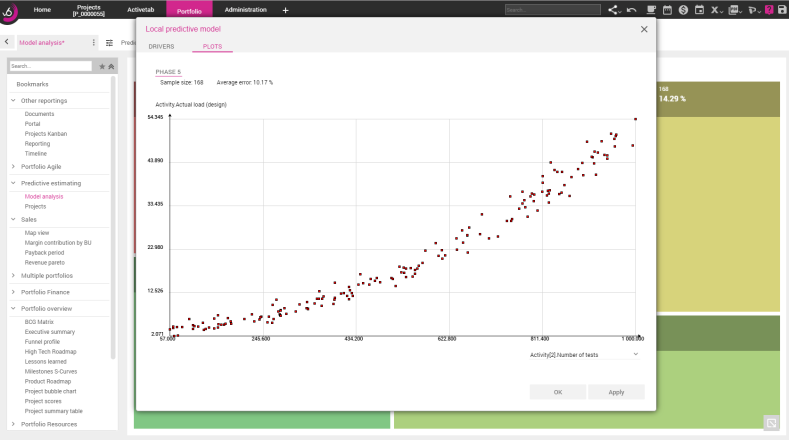
Above: Building and training the predictive model
“Usually the best ideas are not coming from us but from our user base. We are still early on and continue to explore the benefits of using Machine Learning with our customers. We provide cool tech,” concludes Pierre. “But then our customers show us what can really be done with it.”